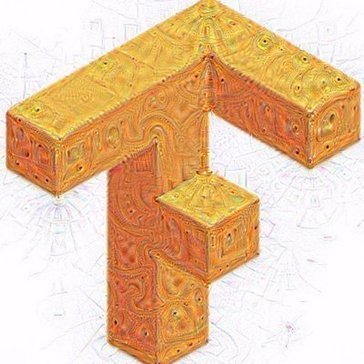
TensorFlow
TensorFlow provides a versatile open-source platform for machine learning, enabling users to effortlessly create, train, and deploy models across various environments. With intuitive APIs, interactive examples, and a robust ecosystem, it supports advanced research and practical applications, from traffic forecasting to playlist generation, while promoting collaboration and educational resources.
Top TensorFlow Alternatives
Universal AI
Lexopedia AI is an advanced machine learning software revolutionizing research and knowledge discovery.
TradeEdge Data Harmonization
TradeEdge Data Harmonization transforms disparate external business data into actionable insights for enterprises.
Eternity AI
Eternity AI's HTLM-7B model harnesses real-time internet knowledge to generate human-like responses.
Segmind
Segmind is a cutting-edge machine learning software that empowers users to harness advanced AI models effortlessly.
DeepLobe
DeepLobe enables users to transform images, text, or videos into actionable insights effortlessly.
3D Measure Up
3D Measure Up revolutionizes measurement accuracy in engineering projects by offering precise 3D visualization and real-time data integration.
PredictSense
It empowers businesses to unlock and monetize their data investments efficiently...
Saturn Cloud
With support for any framework and seamless integration, teams can quickly prototype ideas and transition...
scikit-learn
Its modular design allows users to easily create complex data analysis workflows, making it indispensable...
RapidMiner
With tools for predictive modeling, real-time insights, and intelligent automation, it modernizes existing systems and...
BigML
By standardizing the process, it reduces dependency on complex libraries, facilitating efficient predictive solutions across...
Azure Machine Learning Studio
This service streamlines the development process, enabling efficient experimentation and deployment of predictive analytics solutions...
Google Cloud AutoML
Utilizing advanced transfer learning and neural architecture search, it features a user-friendly graphical interface for...
Simplismart
Users can efficiently run parallel training experiments, monitor resource utilization, and leverage custom or open-source...
machine-learning in Python
Users can easily deploy it using either Rancher or Docker Compose, ensuring flexibility across different...
TensorFlow Review and Overview
Integrating machine learning models into any program is a pain for most developers. There are several third-party libraries that should be included within the project source for the system to work. This creates a lot of confusion among the developers and may result in the incomplete implementation of such code. There are some frameworks that do the job of machine learning integration efficiently without any extra steps. TensorFlow is such a collection of library files that facilitate the use of artificial intelligence and machine learning models within a program. It is based on several APIs that also help to extend the functionality of the program. TensorFlow helps in building customizable neural networking setups and train them as intended.
Suitable for both beginners and expert users
TensorFlow makes it easy for both a beginner developer and an advanced coder. Both of them can use different methods to implement a logic using the TensorFlow library. Beginners are provided with a sequential API, which is more user friendly and is less complicated compared to the advanced setup. Machine learning models can be created by just using the building blocks of code provided by the TensorFlow API. They can import the Tensor modules into the source code and then start using them directly.
Experts can use TensorFlow to fiddle with commands and methods and configure them to their liking. They are provided with the Subclassing API that enables better manual control over the configuration settings. It allows a define-to-run approach for more advanced runs. Easily create classes and forward the sequences imperatively to make it work. Perform custom loop generation and activations and run them as they wish.
The Keras API and its usage in TensorFlow
It uses the Keras API for more advanced and high-level API configurations. It remains as the standard for developing efficient and fast neural networks with better handling of data. Keras API enables speedier prototyping and research about the data supplied to them. The output production and analysis of data are also useful when using Keras API.
Keras has two different modes of importing and execution – a sequential model and functional API, which performs tasks in a different manner. The sequential model is more suited for situations where there is precisely one input Tensor and an output Tensor. The processing is also done sequentially. The functional API provides ways to develop much more flexible models than the sequential API. It can handle layers with non-linear topology and also process multiple channels of inputs and outputs.
Accelerators and estimators in TensorFlow
Processing machine learning and artificial intelligence models require a substantial amount of system resource usage. It can become difficult for the system to handle the processing of such algorithms if they are not efficiently dealt with. The TensorFlow application carefully manages the load by shifting the process into hardware-accelerated mode by using the Graphics processor to compute such tasks without being resource-heavy.
Estimators are a different kind of API that facilitates parallel processing and represents a complete model as a high-level API. It is designed for scaling the code and project while also performing asynchronous training. The data input pipelines in TensorFlow allows the user to input complex data from reusable pieces.
The TensorBoard is a set of data visualization tools to understand and debug the programs developed using the TensorFlow library. There are structured neural inputs to train existing neural networks using structured signals.
Top TensorFlow Features
- Intuitive high-level APIs
- Interactive code samples
- Client-side model execution
- Pre-trained models for deployment
- Comprehensive resource library
- Production ML pipelines
- Domain-specific application packages
- Browser-based model training
- Edge device deployment
- Data preprocessing capabilities
- Model visualization tools
- Performance optimization tools
- Collaborative developer community
- Curated machine learning curriculums
- Standard datasets collection
- MLOps best practices implementation
- Model evaluation tools
- Real-time model tracking
- Multi-language API support
- Continuous updates and announcements